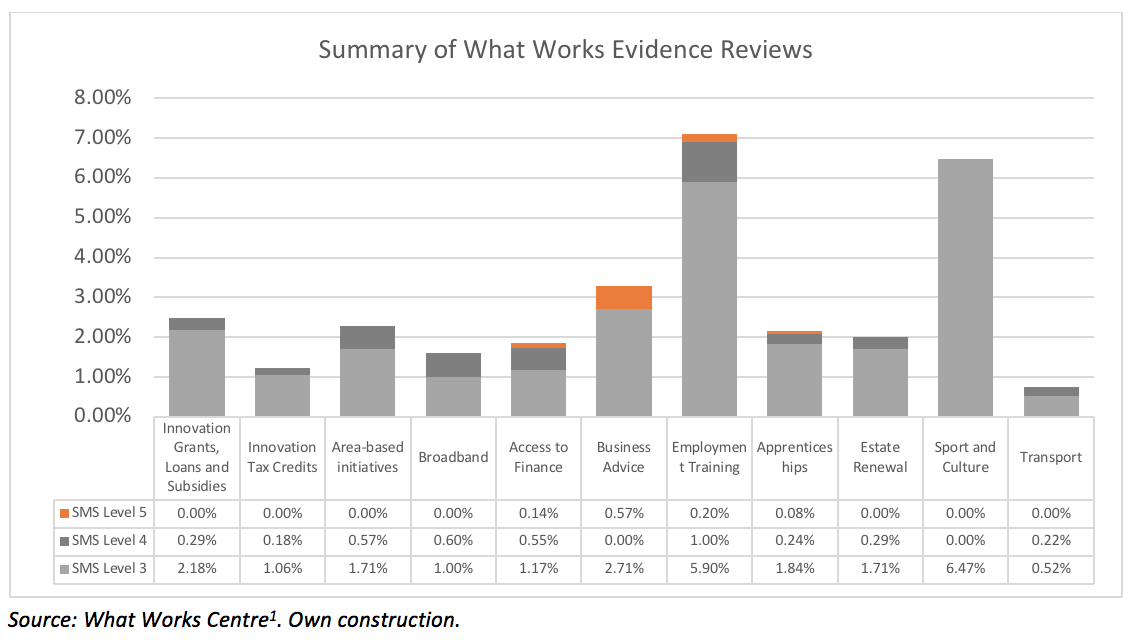
Blog
Using experimental evidence for policymaking: comparative analysis of results obtained from experimental and non-experimental methods in the IEG field
1 October 2020
By Matias Grau Veloso; Hans Frech La Rosa; Lucia Perez Alfaro; Francisca Rabanales; Kathryn Reberg
Despite the importance of entrepreneurship and small and medium enterprises (SMEs) to economic growth, very little policy supporting the growth of these industries is subjected to rigorous evaluation.
Without the use of rigorous evaluation, policymakers are unable to make informed decisions, and risk making ineffective and inefficient use of limited public funds. However, not all evidence is created equal, and knowing the merits of different evaluation strategies is imperative to making evidence-based policy decisions. While there is an abundance of research on various evaluation methodologies, and an increasing number of initiatives working to increase the evaluation capacity of policymakers, there exist no comparative analyses highlighting the difference of evaluation strategies in this policy field. Thus, what are the differences in outcomes between different evaluation strategies? Why do these differences occur? And how do they affect policy decision-making?
Randomised controlled trials (RCTs), have long been thought of as the evaluation gold standard, but represent a miniscule share of evaluations in the IEG field. A breakdown of Maryland Scale ratings of evaluations for common IEG policies found that very few reached a Level 5 – where evaluations were conducted with the method with the highest ability to estimate a causal effect, RCTs.
Figure 1: Summary of Evidence Reviews by SMS Level
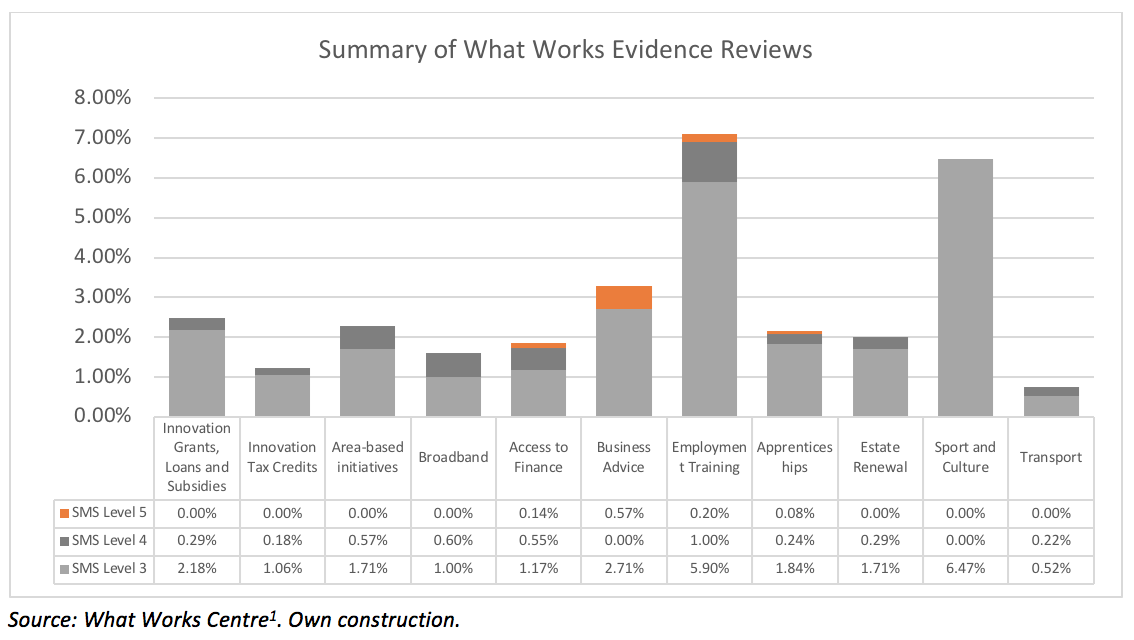
We conducted two comparative case-based quantitative analyses. In these, data from completed IEG RCTs was treated as if no randomisation had occurred and common non-experimental methods were applied. Potential cases were assessed, and ultimately selected, based on the applicability and relevance of the original study, as well as the feasibility of conducting non-experimental methods with the data.
The first case analysis utilises data from the GATE experiment, which provided subsidised entrepreneurship training within the United States between September 2003 and July 2005. Using an oversubscription RCT design, a training subsidy was randomly assigned and business creation, monthly sales, number of employees, and household income were measured in the short-, medium-, and long-term. The RCT finds that subsidised training increased the likelihood of business ownership by 13 per cent in the short-term but finds no other statistically significant effects of the programme. Thus, results from the RCT provide little evidence that the performance of businesses created by those who receive subsidised training are more successful than those who do not. These results were compared with four non-experimental methods: OLS regression, Difference-in-Differences, Propensity Score Matching with internal data, and Propensity Score Matching with an external data source. As can be observed from the following graph, we find that selection bias leads to an overestimation of the impact of subsidised training, whereby people who decide to take-up the offer of subsidised training have different characteristics than those who do not, making them more likely to succeed.
Figure 2: Results of Experimental and Non-experimental Methods in the GATE Case Study
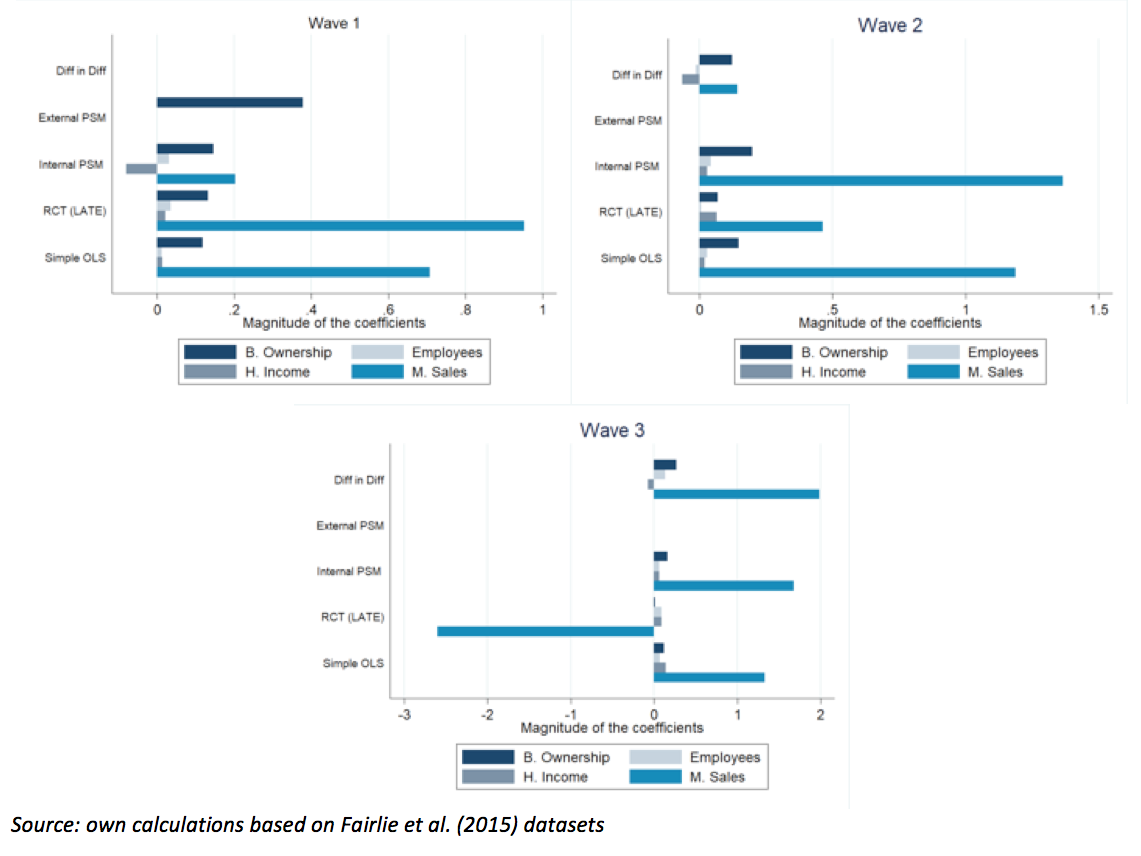
The second case analysis utilises data from Nesta’s Creative Credits experiment, a pilot programme conducted in the Manchester City region in 2009-2010. Here, SMEs were randomly allocated vouchers to pay for creative services, and their effects on innovation measures –introduction of product or service, new to the market and process innovations – were evaluated. The RCT finds that SMEs that received the credit were more likely to have introduced innovations in the six months following the end of the creative projects, but that the credit had no effect after twelve months. Similarly, the RCT results help illuminate the direction of the causal relationship between creative services and innovation. These results were compared with two non-experimental methods: a before and after comparison, and Panel Data. From the following table, we can establish that the non-experimental methods studied underestimate the effect of the Creative Credit on innovation due to time-variant characteristics (for example, the recession that occurred during that period) not controlled for in the models.
Figure 3: Effect of Being a Creative Credit Recipient on the Likelihood of Introducing Each Type of Innovation – RCT vs. Panel Data
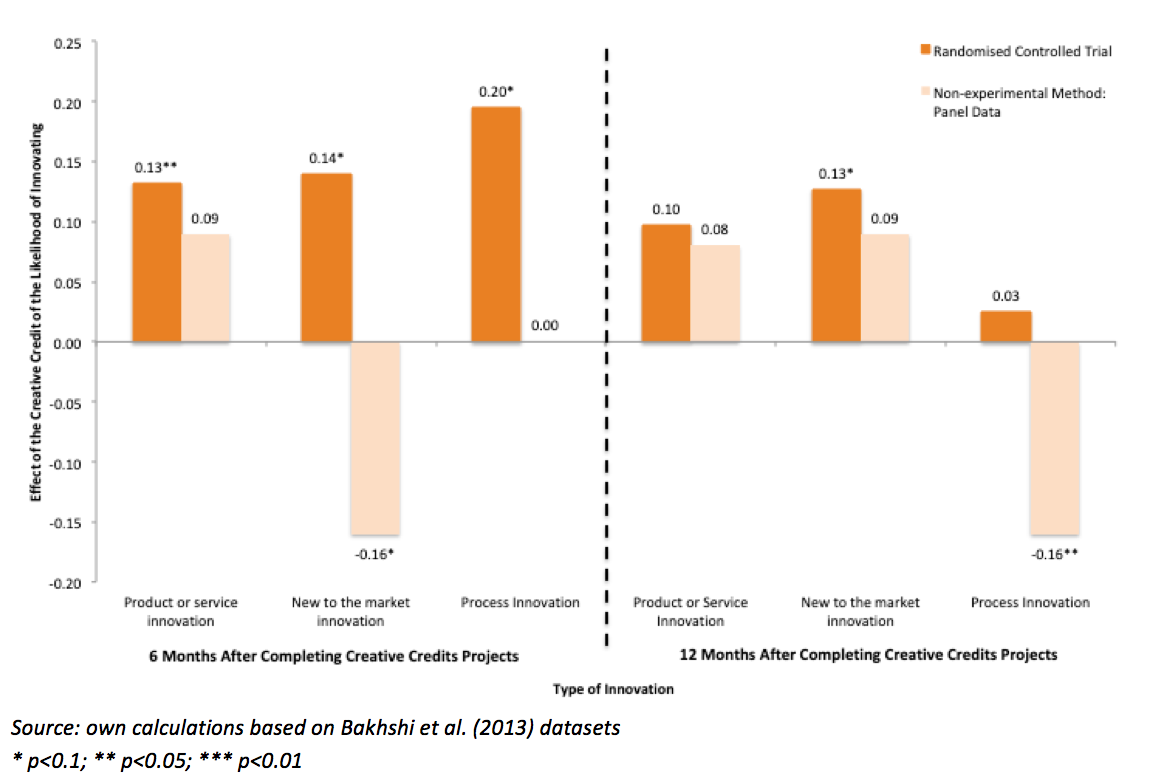
In both cases we find that non-experimental methods are plagued with biases and provide drastically different results than those obtained from the RCT, leading to four clear conclusions for IEG policymakers.
First, in comparing the results obtained from non-experimental and experimental methods, we see that the former cannot be used to estimate the causal effect of a policy. Second, there is no evidence that there exists a non-experimental method that consistently works best in all cases. The “best” of these methods will depend on the scenario and specifics of each case; demonstrating how difficult it is to generate reliable evidence for accurate policy decision-making. Third, without an RCT for comparison, it is impossible to know the magnitude of selection bias present in non-experimental methods. Fourth, the results of non-experimental methods are sensitive to the structure of the database and the structure of the model.
Without knowing what works, policymakers are left guessing what policies to implement. When these decisions are based on non-experimental evidence, policymakers are prone to make investments that are ineffectual and inefficient. The difference in results between RCTs and non-experimental methods, illuminated by our two case studies, highlight the cost-benefit implications of these differing evaluation methodologies. Overestimation of results can lead to policymakers having outsized expectations of success and investing in policies that won’t actually have impact. On the other hand, the underestimation of results can lead to pre-emptive cancellation of programmes, and a failure to invest in policies that would be successful. Thus, while cost is an oft-cited barrier to RCTs, in not implementing them policymakers spend resources on programmes that do not have any impact, or not spend resources on programmes that do have an impact and hence provide a possibility of increasing revenues. Due to these issues, the cost of not using experimental methods could be larger than those of implementing RCTs.
[1] What Works Centre for Local Economic Growth. https://whatworksgrowth.org/policy-reviews/ (Accessed 07 May 2019)